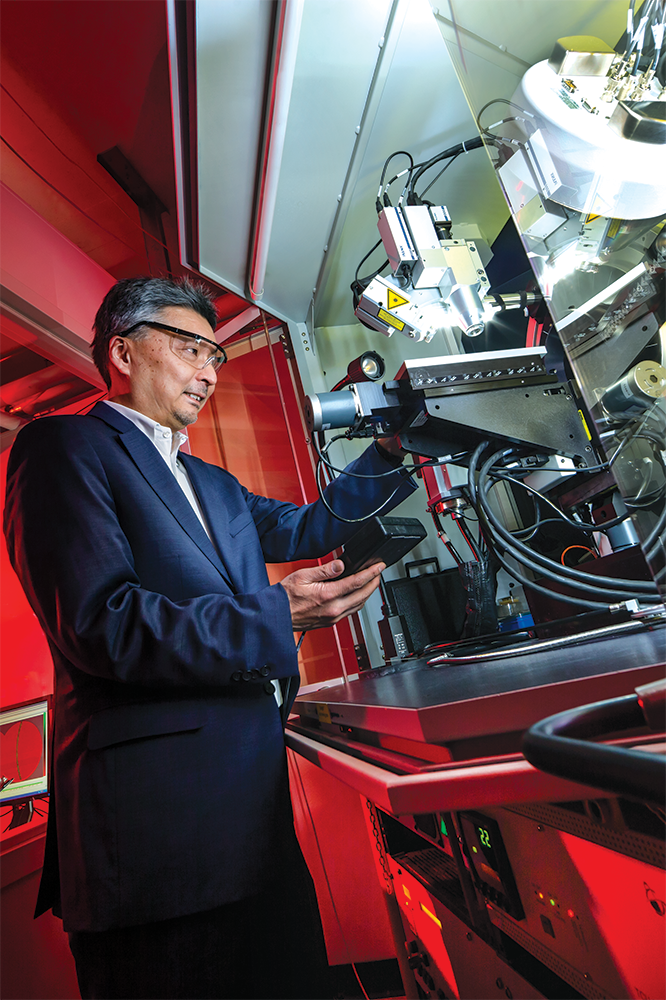
|
 |
Professor Ichiro Takeuchi pictured with an X-ray diffractometer. Photo: John T. Consoli |
|
More than a decade ago, Ichiro Takeuchi, professor of materials science and engineering, started applying the subfield of artificial intelligence (AI) known as machine learning (ML) to help develop new magnetic materials.
At the time, ML was not widely used in materials science. “Now, it’s all the rage,” says Takeuchi, who also holds an appointment with the Maryland Energy Innovation Institute. Its current popularity is due in part to the deep learning revolution of 2012 and related advances in computer chip speed, data storage options, and rapid refinement of the science that drives its predictive analytics of algorithms.
ML-based discovery in materials science is not just a lab exercise. It can provide production solutions to geopolitical challenges—as in the case of deteriorating trade with China about a decade ago, which prompted a supply-chain crisis for electric vehicle motor development in the U.S. Key materials were no longer available to American producers to make the neodymium rare-earth permanent magnet that helps power the vehicles.
The solution: Takeuchi’s team applied ML to discover and develop new, alternative magnet materials so research for electric vehicle motors could continue.
And they bootstrapped it. In the beginning, Takeuchi and his team didn’t have any curated data to feed their ML algorithm. So they built the database themselves. They taught machines to read troves of scientific papers and parse data in search of patterns and predictions. From those papers, they extracted meaningful chemical details on rare-earth magnet performance, properties, and functions. This became the database they needed to enlist the aid of yet another ML algorithm. This time, the task was to identify alternative candidate materials with the desired traits for fabricating rare-earth permanent magnets.
According to Takeuchi, researchers increasingly search for novel materials with specific attributes. “ML helps us in our searches in a way that is computationally inexpensive and highly efficient, so we can understand composition–structure relationships and functional properties.’’
In Takeuchi’s lab, searches for new materials are done with accelerated synthesis of large numbers of compounds called high-throughput experiments, which produce up to 1,000 materials at a time and generate immense quantities of data. “We were inundated with data,” Takeuchi says. Yet prior to applying ML, they lacked a means for leveraging of all that data potential.
ML not only makes sense of enormous datasets—it extends discovery by allowing the algorithm to make predictions from “leads” it discovers in the data. The machine automatically discovers hidden relationships between materials and their properties, which is the knowledge Takeuchi and his team are ultimately seeking.
Takeuchi’s lab continues to innovate with ML-based discovery. Their newest development sprang from the question: “In the search to discover new materials with particular attributes, why don’t we let the computer analyze all the attributes and decide how the experiment should run?”
This new model of autonomous active learning is fast, inexpensive, and highly efficient, because the power and predictive ability of ML minimizes the number of experiments required to solve a problem.
“With an autonomous active learning approach, you don’t need to do 1,000 experiments as we did with high-throughput approaches,” Takeuchi says. “We need only to do about one-tenth or one-fifth of all experiments, because we let the algorithm decide where to go next. You see what the machine comes up with—without you. It predicts, and then we test. We think this is the future.”
Related Articles:
The Battery Revolution Machine Learning's Translational Medicine The Buddy System: Human-Computer Teams Measuring Change in the Atmosphere Clark School Faculty Receive CAREER Awards Study validates face recognition experts, but shows humans perform best with an AI partner MATRIX Lab Hiring Research Development Director Maryland Engineering to Highlight Educational Advances at the 2024 ASEE Annual Conference Dutt is PI on NSF-Funded Quantum Research Grant Maryland Engineers Awarded Grants to Address Humanity's Grand Challenges
April 11, 2019
|